Xiangyu Zhang (Purdue)- Reducing LLM Hallucination in Program Analysis Tasks
Abstract: In this talk, I will present our recent efforts in reducing LLM hallucination in program analysis tasks such as decompilation, data-flow analysis, and bug finding. Although many have started to use LLMs and Code-Language models in program analysis and program transformation tasks, the results haven’t met our expectations. The reason is that these large models hallucinate a lot in complex tasks. There are various reasons behind this. For example, these models treat programs no different from natural language texts during pretraining, although the former have a fundamentally different nature (e.g., due to loops, recursions, and modular design). In addition, the models usually have limited input sizes, which are insufficient for complex tasks. I will present a few methods we have developed to reduce hallucination in program analysis, including a novel pre-taining method that challenges the model to understand program semantics by understanding data-flow, a novel context propagation method that addresses model input limits, and a new end-to-end LLM based bug detection pipeline that does not directly prompt the LLM to find bugs, but rather requests the LLM to synthesize code to perform deterministic detection and result sanitization.
Speakers
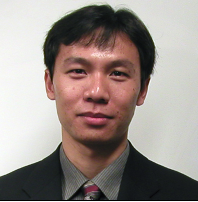
Xiangyu Zhang
Xiangyu Zhang is a Samuel Conte Professor at Purdue specializing in AI security, software analysis and cyber forensics. His work involves developing techniques to detect bugs, including security vulnerabilities, in traditional software systems as well as AI models and systems, and to leverage AI techniques to perform software engineering and cybersecurity tasks. He has served as the Principal Investigator (PI) for numerous projects funded by organizations such as DARPA, IARPA, ONR, NSF, AirForce, and industry.