Quantum Materials, Built By AI Robot
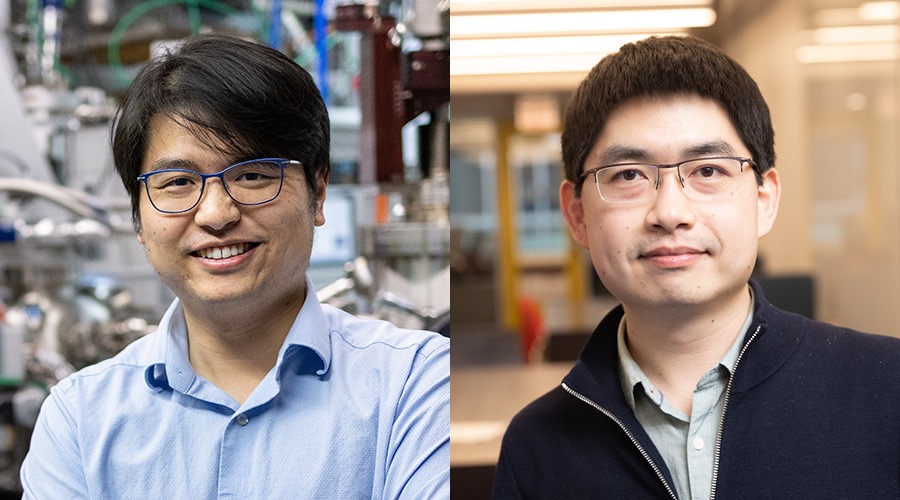
Atomically thin superconducting quantum materials extending to wafer scales are some of the most important, yet hard to fabricate, materials in the quantum world.
“Within the US, there are fewer than 10 research groups that have successfully fabricated high-temperature iron selenide superconductors,” said Asst. Prof. Shuolong Yang of the University of Chicago Pritzker School of Molecular Engineering (UChicago PME). “This is an art. It’s not only about the fascinating quantum physics but also about craftsmanship.”
Buoyed by a $500,000 National Science Foundation grant, Yang and UChicago Computer Science Asst. Prof. Yuxin Chen are developing self-learning AI robots to fabricate quantum materials quickly, efficiently and without human intervention.
Working at the intersection of quantum science, materials science and AI-driven manufacturing, this research could put more and more types of quantum materials in the hands of researchers and manufacturers across the globe, opening the door for future innovations, insights and technological breakthroughs.
“This has the potential to ensure an important quantum technology will be accessible to a bigger research and industrial community,” Yang said.
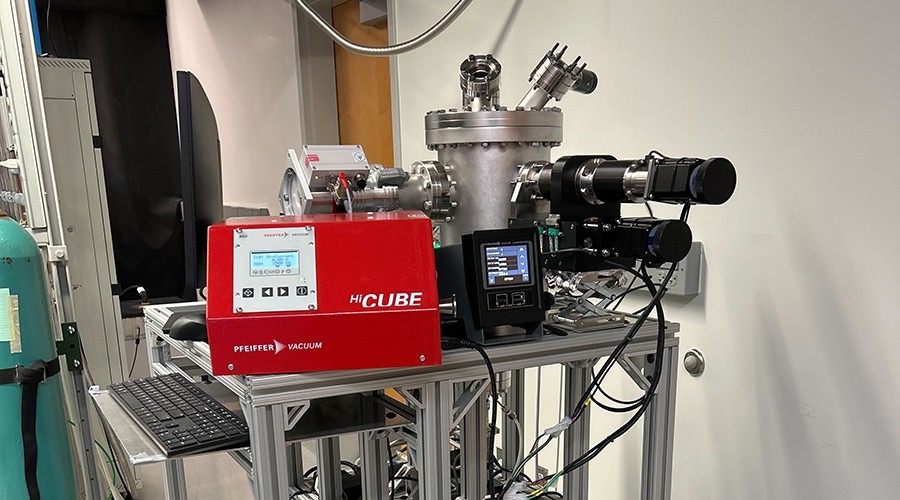
AI, Robot
Combining UChicago PME’s material science and quantum expertise with the Computer Science Department’s AI and machine learning acumen, the team hopes to automate and improve the molecular-beam epitaxy (MBE) process, one of the ways researchers grow material an atomic layer at a time.
Chen called the project “a two-way street” that should improve both MBE and AI.
“We have this suite of AI algorithms, which we believe could be used as baselines to demonstrate that AI can help with MBE. In turn, the MBE challenge inspires us to develop new or more sophisticated AI techniques,” he said.
MBE involves checking and rechecking diffraction patterns to make sure the material is growing the way the researchers intend. Researchers use historical data, experience and sometimes gut instinct to adjust conditions along the way to fix problems as they arise.
“If you train a human to do it, it often takes years to actually develop and internalize this craftsmanship for thin-film growth,” Yang said.
Based on their previous successes building an AI robot to deposit the thin layers of silver for optical beam splitters, the team hopes to develop that craftsmanship in machinery.
“We need to enable the AI to make real-time recommendations, real-time decisions to guide the design of the experiments,” Chen said. “The need for instant adaptive adjustment also pushes machine learning towards faster, more efficient, in our case, reinforcement learning algorithms.”
Jumping in the deep end
Quantum materials are finicky. Even the slightest error will make the material unsuitable for quantum computing, communications, sensing or other applications.
“Historically, people have relied on experienced hands and eyes to fabricate such superconductors,” Yang said. “We’re trying to systematically combine artificial intelligence and thin-film growth technologies to create a robot to fabricate these important superconducting materials.”
To test the AI robot concept on quantum materials, the team is jumping in the deep end, focusing on monolayer iron selenide superconductors, a tricky material to fabricate even by quantum standards. Even the most talented researchers can make one to two of such thin-film superconductors a day, Yang said.
This will make it difficult to generate enough data to train the AI, Chen said. To overcome this data gap, the team is using a reinforcement learning model, rather than a purely supervised learning approach like the labeled image datasets used for CAPTCHA or the initial training phase of large language models.
Supervised learning will teach an AI what a cow is, for example, by feeding it thousands upon thousands of images of cows labeled “cow.” Over time, the AI will learn what is and what is not a cow.
Reinforcement learning teaches AI to maximize a specific reward. Rather than flooding it with data on ideal quantum materials – which are a lot harder to come by than photos of cows – it focuses on finding what materials work best.
This uses the AI itself to filter the better superconductors from the lower-performing ones. The AI uses the best results to teach itself how to find even better ones, opening up new quantum materials, techniques and possibilities.
“The goal is to train a model that can ask what data is most useful for improving itself,” Chen said, “and eventually become better at guiding the design of experiments.”
This story was written by Paul Dailing and posted originally to the PME website.